It seems that almost every week, there is a new headline about someone committing fraud and stealing from their employer. While every fraud is unique, identifying patterns can help organizations better understand how and why fraud happens. There are several fact patterns that professionals at Forvis Mazars have noticed recently in frauds involving accounts payable:
- Employees working together to circumvent internal controls
- Depositing stolen funds into accounts controlled by the perpetrator(s)
- Fictitious vendors and invoices
Organizations with proper separation of duties and other preventive internal controls still need detective internal controls (which aim to detect fraud that already occurred)1 due to potential collusion. Here, we lay out the impact of collusion on fraud and how data analytics can help.
Collusion Muddies the Waters
Employees collaborating to circumvent internal controls—also known as collusion—can make fraudulent activity difficult to detect. Even the most well-rounded internal control system with proper separation of duties in place must, at some point, rely on employees to be honest.2 In the 2022 Report to the Nations, the Association of Certified Fraud Examiners (ACFE) found that a higher number of perpetrators increases the median losses suffered by organizations.3
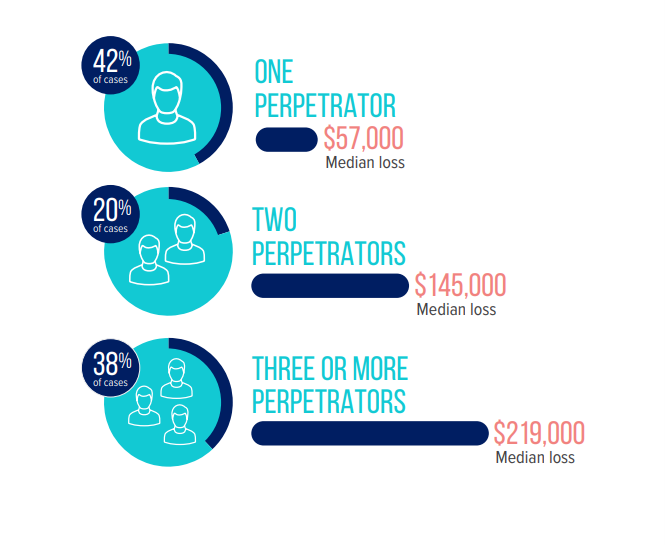
A proper separation of duties introduces a monitoring function, as another person verifies each person’s actions. However, if collusion exists among those persons, a company will be vulnerable to fraud. We have seen cases where employees have colluded by having one employee prepare checks written to fake vendors with fake addresses and another employee intercept those checks once they are signed. This second step was crucial as the checks would have been undeliverable with a fake address. In this situation, the employee who intercepted the checks then deposited the checks into a bank account they controlled. The profits were then shared between the perpetrators.
The threat of collusion shows us that the perception of strong internal controls and constant monitoring can be an effective strategy to discourage potential fraudsters’ perception of opportunity. However, this perception means little without effective detective and preventive measures in place.
Accounts Payable Data Analytics
Data analytics is an effective detective control to help identify potential fraud in accounts payable. According to the ACFE’s 2022 Report to the Nations, automated transition/data monitoring can help reduce fraud duration to six months.
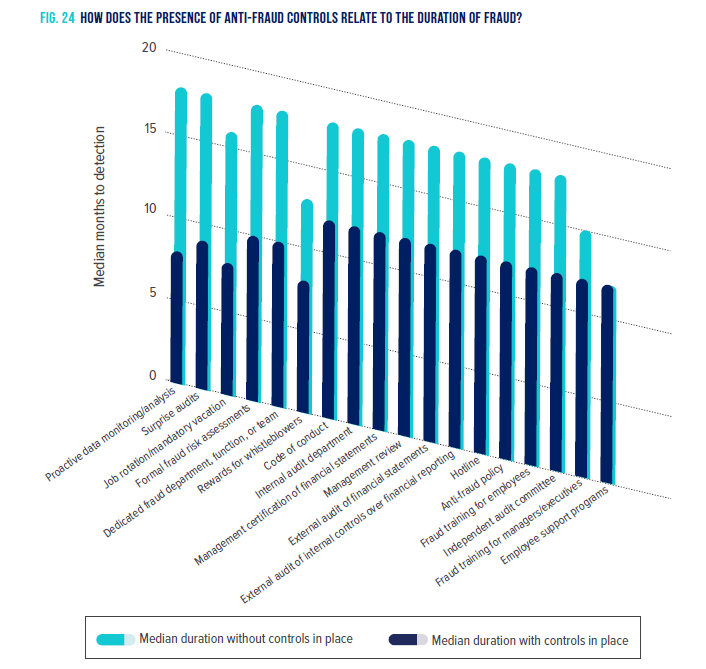
There are several different data analytics techniques that can be used to help identify fictitious vendors and invoices to help implement proactive data monitoring:
- Searching for potential duplicate invoices
- Graphing payments to vendors over time to help identify payment trends
- Looking for shared attributes between employees and vendors
When looking for potential duplicate invoices, it is important to look for things that are similar, but not exactly the same. Depending on the accounting system, there may be configurations to prevent an exact duplicate from being entered, which enforces the need to look for items that are similar. Something to look for are invoices from vendors with slightly different names—sometimes fraudsters will create a fictious vendor that is a slight variation of a legitimate vendor. Another common pattern to look for is similar invoice numbers—sometimes an additional character will be added to an invoice number to mirror a legitimate invoice.
Graphing payments to vendors over time provides great operational insights and visibility into unusual payment patterns to vendors. A common pattern to see in a fraud investigation is an acceleration pattern. In this pattern, a vendor utilized for fraudulent activity will initially receive small payments. If these small payments go unnoticed, the perpetrator(s) will increase payments over time. This pattern can be seen visually in a graph.
Depending on the sophistication level of the perpetrator(s), they may create a fictitious vendor that shares some of their personal attributes such as address or bank account information. Looking for employees who share attributes with vendors can help identify potential fictitious vendors or undisclosed relationships between employees and vendors. When performing a procedure like this, it is important to standardize data as much as possible prior to matching because there can be variations in the data that make matching difficult. Another technique to consider using is a fuzzy matching process to identify similar matches.
These are just a few examples of data analytics techniques to help identify fraud in accounts payable. Implementing these procedures—or similar analytics procedures—on a recurring basis can be an effective detective control. Through our experience, a combination of strong preventive and detective controls creates a more robust environment to help fight fraud.
If you have any questions or need assistance, please reach out to a professional at Forvis Mazars.
- 1“Auditing Standard No. 5, An Audit of Internal Control Over Financial Reporting That Is Integrated with An Audit of Financial Statements, Appendix A - Definitions,” pcaob.org, 2023.
- 2 a b c “Fraud Examiners Manual,” 1.441, acfe.com, 2022.
- 3“Occupational Fraud 2022: A Report to the Nations®,” legacy.acfe.com, 2023.